Toolchains¶
A toolchain describes the workflow of a particular experiment. Its declaration defines:
- a collection of processing blocks, including for each of them:
- a name (unique in the toolchain)
- a list of inputs
- a list of outputs
- the interconnections between those blocks (from an output to an input)
- a list of datasets, that yield raw input data for the experiment
- a list of result outputs, that produce the results of the experiment
Declaration of a toolchain¶
Note
One needs only to declare a toolchain using those specifications when not using the web interface (i.e. when doing local development or using the web api). The web interface provides a user-friendly way to declare and modify toolchains.
A toolchain is declared in a JSON file, and must contain at least the following fields:
{
"datasets": [
],
"blocks": [
],
"connections": [
],
"analyzers": [
]
}
Note that this toolchain is considered as a correct one by the platform (i.e. it doesn’t contain any error, and thus can be modified via the web interface), but not as an executable one, as there is nothing to execute.
For display purposes, the JSON file may contain an additional field called representation, which provides insight on how to display the workflow in a graphical way.
Declaration of the datasets¶
Datasets are starting points of a toolchain. They provide raw input data for a scientific experiment, and they have, hence, outputs but no input. Several datasets are typically attached to a given a protocol of a database, each of them having a particular role. For instance, a protocol for a classification task may provide three distinct datasets, one for training a generic model, one for enrolling class-specific models, and one for generating probe samples that are compared against the enrolled models.
To define the dataset, its name as well as its corresponding outputs have to be defined in the JSON declaration of the toolchain. Considering the example mentioned above, this would look like:
{
...
"datasets": [
{
"outputs": [
"image",
"eye_centers"
],
"name": "train"
},
{
"outputs": [
"template_id",
"client_id",
"image",
"eye_centers"
],
"name": "templates"
},
{
"outputs": [
"probe_id",
"client_id",
"template_ids",
"image",
"eye_centers"
],
"name": "probes"
},
...
}
Declaration of the processing blocks¶
To define the processing blocks contained in a toolchain, just add some entries
into the blocks
array, such as:
{
...
"blocks": [
{
"inputs": [
"image",
"eye_centers"
],
"synchronized_channel": "train",
"name": "cropping_rgb_train",
"outputs": [
"image_gray"
]
}
]
...
}
Here we defined a block named cropping_rgb_train, which expects two inputs, image and eye_centers, and returns one output, image_gray. The synchronization channel indicates against which dataset the outputs are synchronized. However, the toolchain does specify neither the data format of the inputs and outputs, nor the algorithm that is going to run inside the block. This is performed in the experiment definition, which combines dataformats, algorithms and a toolchain together.
As with the datasets, to define more blocks just add more entries into the
blocks
array:
{
...
"blocks": [
{
"inputs": [
"image",
"eye_centers"
],
"synchronized_channel": "train",
"name": "cropping_rgb_train",
"outputs": [
"image_cropped"
]
},
{
"inputs": [
"image_cropped"
],
"synchronized_channel": "train",
"name": "feature_extraction_train",
"outputs": [
"feature_vector"
]
}
]
...
}
Declaration of the connections between the processing blocks¶
To define a connection between two processing blocks (or one dataset and one
processing block), just add one entry into the connections
array, with one
of the following forms:
{
"from": "block1_name.output_name",
"to": "block2_name.input_name"
}
or:
{
"from": "dataset_name.output_name",
"to": "block_name.input_name"
}
For example:
{
...
"connections": [{
"from": "cropping_rgb_train.image_cropped",
"to": "features_extraction_train.image_cropped"
}
],
"blocks": [
{
"inputs": [
"image",
"eye_centers"
],
"synchronized_channel": "train",
"name": "cropping_rgb_train",
"outputs": [
"image_cropped"
]
},
{
"inputs": [
"image_cropped"
],
"synchronized_channel": "train",
"name": "feature_extraction_train",
"outputs": [
"feature_vector"
]
}
]
...
}
Several important things to note:
- The names of the connected output and input don’t need to be the same. Use whatever make sense in the context of each block
- An output can be connected to several inputs
- An input can only be connected to one output
- The names of the blocks and of the datasets must be unique in the toolchain
Declaration of the outputs to use as results¶
To declare that a particular processing block output produces the result of the
toolchain (or a part of it), just add one entry into the analyzers
field,
with the following form:
{
...
"analyzers": [
{
"inputs": [
"scoring_dev_scores",
"scoring_test_scores"
],
"synchronized_channel": "probes",
"name": "analysis"
}
]
...
}
The field inputs lists the results, while the field synchronized_channel indicates the dataset against which to automatically perform the loop as for any regular toolchain block.
The data written on those inputs will be used to display results and plots on the web interface.
Putting it all together: a complete example¶
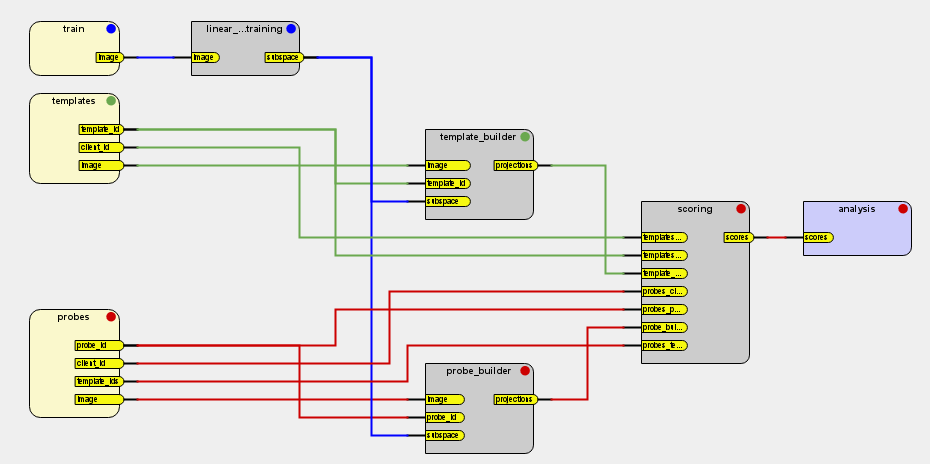
Fig. 8 A complete toolchain that train and test an Eigenfaces system
The following example describes the toolchain visible at Fig. 8, a complete toolchain that:
- train an Eigenfaces face recognition system on one set of images (train)
- enroll client-specific models on another set of images (templates)
- test these models using samples from a third set of images (probes)
Note
A toolchain is still not executable, since it contains no mention of the algorithms that must be used in each processing block, as well as the database to use.
{
"datasets": [
{
"outputs": [
"image"
],
"name": "train"
},
{
"outputs": [
"template_id",
"client_id",
"image"
],
"name": "templates"
},
{
"outputs": [
"probe_id",
"client_id",
"template_ids",
"image"
],
"name": "probes"
}
],
"blocks": [
{
"inputs": [
"image"
],
"synchronized_channel": "train",
"name": "linear_machine_training",
"outputs": [
"subspace"
]
},
{
"inputs": [
"image",
"template_id",
"subspace"
],
"synchronized_channel": "templates",
"name": "template_builder",
"outputs": [
"projections"
]
},
{
"inputs": [
"image",
"probe_id",
"subspace"
],
"synchronized_channel": "probes",
"name": "probe_builder",
"outputs": [
"projections"
]
},
{
"inputs": [
"templates_client_id",
"templates_template_id",
"template_builder_projections",
"probes_client_id",
"probes_probe_id",
"probe_builder_projections",
"probes_template_ids"
],
"synchronized_channel": "probes",
"name": "scoring",
"outputs": [
"scores"
]
}
],
"analyzers": [
{
"inputs": [
"scores"
],
"synchronized_channel": "probes",
"name": "analysis"
}
],
"connections": [
{
"to": "linear_machine_training.image",
"from": "train.image",
"channel": "train"
},
{
"to": "template_builder.image",
"from": "templates.image",
"channel": "templates"
},
{
"to": "template_builder.template_id",
"from": "templates.template_id",
"channel": "templates"
},
{
"to": "template_builder.subspace",
"from": "linear_machine_training.subspace",
"channel": "train"
},
{
"to": "probe_builder.image",
"from": "probes.image",
"channel": "probes"
},
{
"to": "probe_builder.probe_id",
"from": "probes.probe_id",
"channel": "probes"
},
{
"to": "probe_builder.subspace",
"from": "linear_machine_training.subspace",
"channel": "train"
},
{
"to": "scoring.templates_client_id",
"from": "templates.client_id",
"channel": "templates"
},
{
"to": "scoring.templates_template_id",
"from": "templates.template_id",
"channel": "templates"
},
{
"to": "scoring.template_builder_projections",
"from": "template_builder.projections",
"channel": "templates"
},
{
"to": "scoring.probes_client_id",
"from": "probes.client_id",
"channel": "probes"
},
{
"to": "scoring.probes_probe_id",
"from": "probes.probe_id",
"channel": "probes"
},
{
"to": "scoring.probe_builder_projections",
"from": "probe_builder.projections",
"channel": "probes"
},
{
"to": "scoring.probes_template_ids",
"from": "probes.template_ids",
"channel": "probes"
},
{
"to": "analysis.scores",
"from": "scoring.scores",
"channel": "probes"
}
]
}