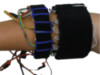
Jaquier, N., Connan, M., Castellini, C. and Calinon, S. (2017)
Combining Electromyography and Tactile Myography to Improve Hand and Wrist Activity Detection in Prostheses
Technologies, 5:4, Special Issue on Assistive Robotics.
Abstract
Despite recent advances in prosthetics and assistive robotics in general, robust simultaneous and proportional control of dexterous prosthetic devices remains an unsolved problem, mainly because of inadequate sensorization. In this paper, we study the application of regression to muscle activity, detected using a flexible tactile sensor recording muscle bulging in the forearm (tactile myography-TMG). The sensor is made of 320 highly sensitive cells organized in an array forming a bracelet. We propose the use of Gaussian process regression to improve the prediction of wrist, hand and single-finger activation, using TMG, surface electromyography (sEMG; the traditional approach in the field), and a combination of the two. We prove the effectiveness of the approach for different levels of activations in a real-time goal-reaching experiment using tactile data. Furthermore, we performed a batch comparison between the different forms of sensorization, using a Gaussian process with different kernel distances.
Bibtex reference
@article{Jaquier17MDPI, author="Jaquier, N. and Connan, M. and Castellini, C. and Calinon, S.", title="Combining Electromyography and Tactile Myography to Improve Hand and Wrist Activity Detection in Prostheses", journal="Technologies", year="2017", volume="5", number="4", pages="1--16", note="Special Issue on Assistive Robotics" }