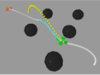
Lembono, T.S. and Calinon, S. (2021)
Probabilistic Iterative LQR for Short Time Horizon MPC
In Proc. IEEE/RSJ Intl Conf. on Intelligent Robots and Systems (IROS), pp. 556-562.
Abstract
Optimal control is often used in robotics for planning a trajectory to achieve some desired behavior, as expressed by the cost function. Most works in optimal control focus on finding a single optimal trajectory, which is then typically tracked by another controller. In this work, we instead consider trajectory distribution as the solution of an optimal control problem, resulting in better tracking performance and a more stable controller. A Gaussian distribution is first obtained from an iterative Linear Quadratic Regulator (iLQR) solver. A short horizon Model Predictive Control (MPC) is then used to track this distribution. We show that tracking a distribution is more cost-efficient and robust as compared to tracking the mean or using iLQR feedback control. The proposed method is validated with kinematic control of 7-DoF Panda manipulator and dynamic control of 6-DoF quadcopter in simulation.
Bibtex reference
@inproceedings{Lembono21IROS, author="Lembono, T. S. and Calinon, S.", title="Probabilistic Iterative {LQR} for Short Time Horizon {MPC}", year="2021", booktitle="Proc.\ {IEEE/RSJ} Intl Conf.\ on Intelligent Robots and Systems ({IROS})", pages="556--562" }