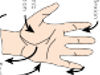
Jaquier, N., Castellini, C. and Calinon, S. (2017)
Improving Hand and Wrist Activity Detection Using Tactile Sensors and Tensor Regression Methods on Riemannian Manifolds
Myoelectric Controls Symposium (MEC).
Abstract
Simultaneous and proportional control of a prosthetic hand and wrist is an ongoing challenge, although giant steps have lately been made in this direction. In this paper, we study the application of a novel machine learning method to the problem, with the aim to potentially improve such control. Namely we apply different kernels for tensor Gaussian process regression to data obtained from an advanced, flexible tactile sensor applied on the skin, recording muscle bulging in the forearm. The sensor is a modular, compact bracelet comprising 320 highly sensitive elements organized as a tactile array. The usage of kernel functions with tensor arguments and kernel distances computed on Riemannian manifolds enables us to account for the underlying structure and geometry of the tactile data. Regression accuracy results obtained on data previously collected using the bracelet demonstrate the effectiveness of the approach, especially when using Euclidean distance and Kullback-Leibler divergence-based kernels.
Bibtex reference
@inproceedings{Jaquier17MEC, author="Jaquier, N. and Castellini, C. and Calinon, S.", title="Improving Hand and Wrist Activity Detection Using Tactile Sensors and Tensor Regression Methods on {R}iemannian Manifolds", booktitle="Myoelectric Controls Symposium ({MEC})", year="2017", month="August", address="Fredericton, New Brunswick, Canada", pages="" }
Source codes
Source codes related to this publication are available as part of PbDlib.